【2018/ECCV】Weakly-supervised 3D Hand Pose Estimation from Monocular RGB Images
TL;DR
在3D hand pose estmation任务中,由于标注的准确性和数量限制,目前方法3D还原效果一般。文中提出了一种RGB和depth image结合的弱监督模型,能够取得不错的效果。
Algorithm/Model
contributions
- Introduce the weakly supervised problem of leveraging low-cost depth maps during training for 3D hand pose estimation from RGB images.
- Propose an end-to-end learning based 3D hand pose estimation model for weakly-supervised adaptation from fully-annotated synthetic images to weakly-labeled real-world images.
- The weakly-supervised approach compares favorably with existing works and the proposed fully-supervised method outperforms all the state-of-the-art methods.
模型如下所示:
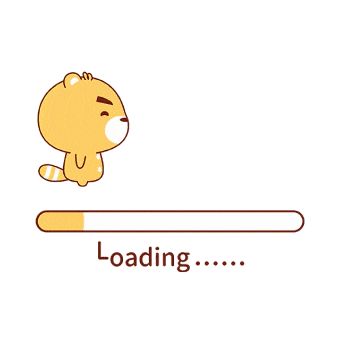
主要的创新点在于将深度图的特征加入模型当中,训练的过程如下所示:
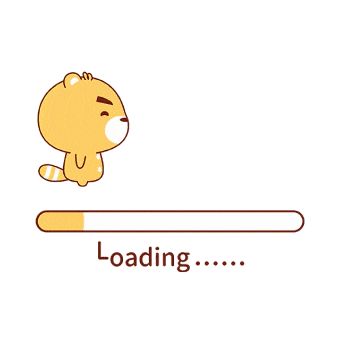
- For weakly-supervised learning, model is pretrained on RHD and then adapted to STB by fusing the training of both datasets.
- For fully-supervised learning, the two datasets are used independently in the training and evaluation processes.
其中depth regularizer结构如下所示:
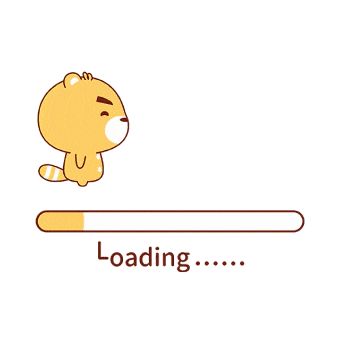
达到的效果如下:
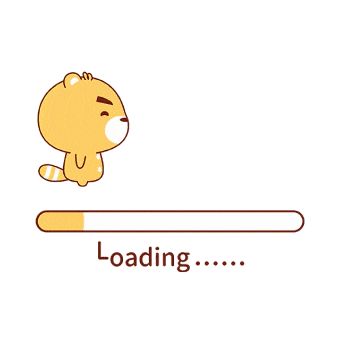
Experiment Detail
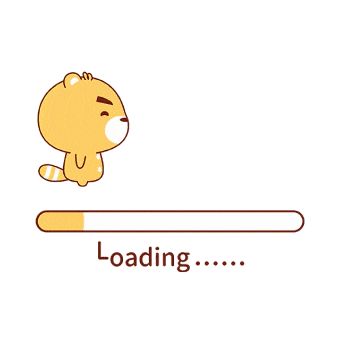
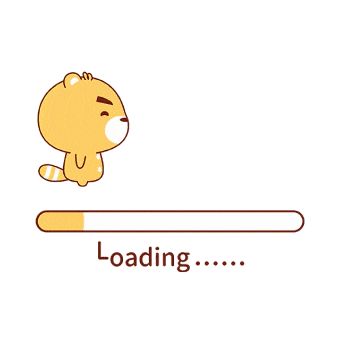
Thoughts
结合深度图是一个不错的想法,但是图片paired这一点限制了模型的泛化能力。
联系作者
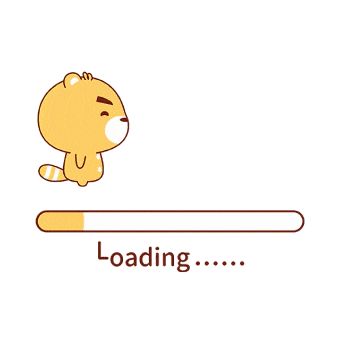
本博客所有文章除特别声明外,均采用 CC BY-NC-SA 4.0 许可协议。转载请注明来自 梦家博客!
评论
TwikooValine